Is Google Analytics 4 a Tactical Move Away From Free Analytics?
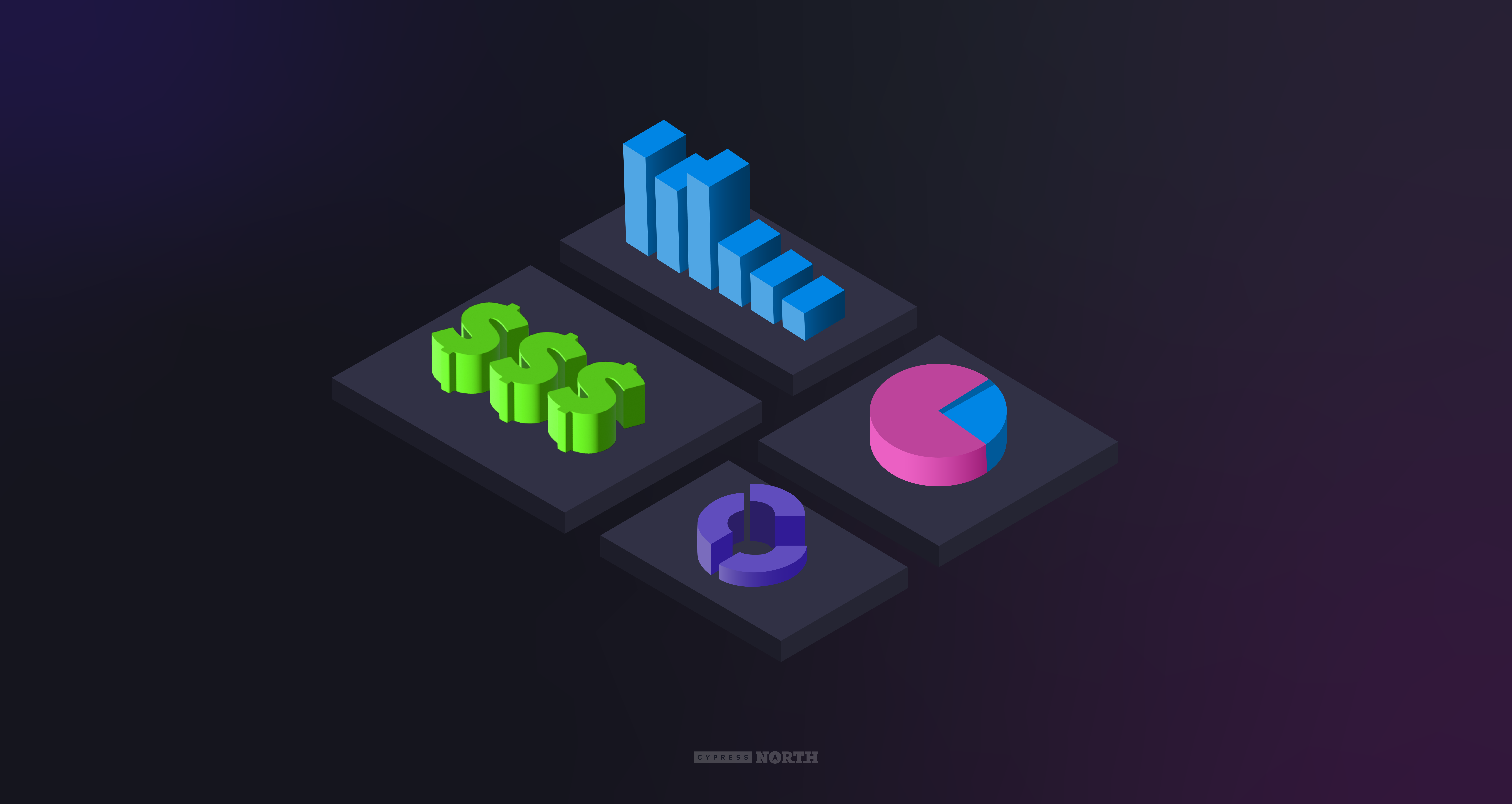
There’s something fishy going on with the way that Google is handling Google Analytics 4. To me, it’s playing out as a backdoor cash grab, hidden under a thin veil of a free and easy migration from Universal Analytics.
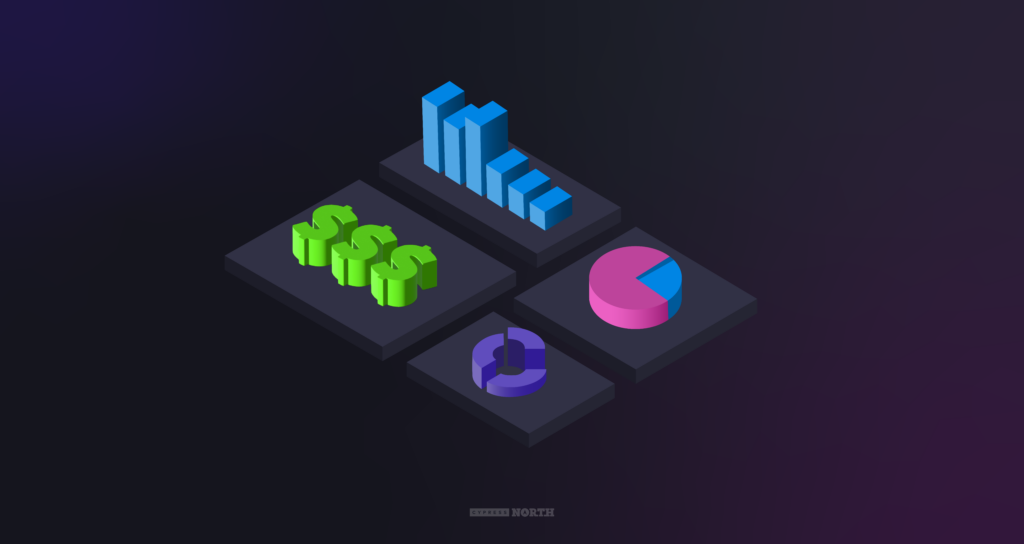
Table of Contents
Google Analytics 4: A Measurable Departure from Previous Analytics Offerings
History: The Sunsetting of UA & Google Analytics 4 The "Next Generation"
A Shift From the Free Analytics Mindset
GA4 Reporting: A Pay-To-Play For Reporting?
API Limits with the Google Ecosystem
Looker Studio & Reporting Solutions in GA4
Trending: A Future With Fewer Statistics
GA4 Reporting: How To See Accurate Data
BigQuery: A Big Expense Needed for Actionable GA4 Use
Technology Without an Audience
Turning All GA4 Data into “Big Data” by Default
Technical and Financial Ramifications
Navigating the Post-UA World
Google Analytics 4: A Measurable Departure from Previous Analytics Offerings
History: The Sunsetting of UA & Google Analytics 4 The "Next Generation"
In the first months after Google announced the sunsetting of UA, we got to work setting up our clients with a GA4 property to go alongside their UA properties. We found that we were able to set up and track most things in a similar way and all seemed like it was going to be fine. During this time we’ve had UA data to fall back on and dozens of reports and dashboards running while UA is still active.
More recently we’ve been working on replacing UA reporting with GA4 reporting for big and small clients alike. What we’re discovering is that all but the simplest and smallest of clients are going to be impacted by this transition in a much more technical, and potentially costly, way than expected.
A Shift From the Free Analytics Mindset
In my role, I’ve been working on the technical side to figure out how to ingest GA4 data into data lakes and make data available for reporting tools. I’ve listened to the problems reported by our marketing team regarding discrepancies in the data tracked in GA4 vs UA which are easy to compare and never the same.
I’ve seen what used to be pretty reliable eCommerce and sales data in UA become all but useless in GA4. And I’ve witnessed Google continue to roll out new restrictions and requirements that have hamstrung our agency’s and our client’s analytics departments.
The picture that is coming together is that Google is done giving analytics away for free. It’s tired of the poor financial performance of BigQuery and Google 360, and at the same time, they have a crippled product thanks to the EU’s data security law and other regional privacy regulations.
GA4 Reporting: A Pay-To-Play For Reporting?
API Limits with the Google Ecosystem
We’ve traditionally put together dashboard reports for our clients using Looker Studio, Tableau, and Power BI depending on the level of the client.
Google's Looker Studio (formerly Data Studio) is the most common since it’s free, and easily accessible. We have a lot of Looker reports connected to UA, some with dozens of pages of reports in them, and it’s always worked well for us. We did this because standard UA reporting was too complicated for our clients to find the information they wanted, and precompiling dashboards for them made their lives easier.
Migrating the reports to GA4 wasn’t super straight forward but it also wasn’t that bad. We were able to recreate them using GA4 data, and despite some early struggles with metrics being wildly different, they improved over time and are now usually about the same.
Still, GA4 data doesn’t exactly match what UA reports for the same time periods which makes cross-comparisons and validation a little tricky. Other metrics have changed dramatically like Time on Page inUA being transitioned to Engagement Time in GA4 which has major differences in definition and what is reported.
That changed quickly when Google announced new API rate limits to GA4 data - even using their own product, Looker Studio.
Looker Studio & Reporting Solutions in GA4
The rate limits are so stingy that any report with more than one or two pages of information would quickly hit the hourly limit and be broken until the next hour. For our enterprise clients who have many people accessing the reports at any time - the reports were broken almost instantly and for all purposes, permanently. What’s worse is that the way basically everybody found out about this was through their broken reports and, ironically, searching Google for a solution.
While it’s true that you can use the standard GA4 reporting inside Google Analytics without these limits, the fact is that the reporting is MILES worse than UA reporting, making a separate reporting tool a necessity.
Trending: A Future With Fewer Statistics
Not only is GA4 reporting worse than UA, but it’s all estimated data!
Google has come out and explained that it’s not feasible for them to process the raw data to provide the metrics we’re all used to - a side effect of their new data format for GA4. Instead, they suggest that the information you see in the GA4 dashboard should be useful for trend guidance and not actual statistics.
That doesn’t sit well with clients who rely on detailed stats for hitting their KPIs and making business decisions.
GA4 Reporting: How To See Accurate Data
So what is Google’s answer for how to report on its own data?
- Buy Google Analytics 360, a $150,000/yr upgrade that has higher rate limits
- Export your raw data to Google BigQuery
The conscious act of A) crippling their standard reporting tools, and B) Implementing an absurdly restrictive API rate limit strikes me as clear efforts to bolster revenue in a long-time loss leader product.
BigQuery: A Big Expense Needed for Actionable GA4 Use
Technology without an Audience
In a typical info-sharing moment at Cypress North, I sent this article from MotherDuck saying “Big Data is Dead” to our Data Analytics team back in February 2023. At the time, it had nothing to do with anything, although I’ve long held the opinions shared in the article that “Big Data” was reserved for a small number of companies in the world.
The TLDR; on this is that a founding engineer of Google BigQuery explains how they created an impressive technical achievement without an audience. Their database system is pretty amazing, allowing for efficient querying of Terabytes of data. The trouble is that almost nobody has that much data to query. Add to that the fact that the rest of the cloud computing world has caught up via raw processing power (or the separation of storage from compute) and you have a technical investment that hasn’t paid dividends.
Turning All GA4 Data into “Big Data” by Default
With the creation of GA4, Google had its perfect “Big Data” product. Compared to UA, the collection of GA4 tracking data is more flexible, and potentially more capable, but hindered by privacy regulations.
In GA4, almost everything is tracked as an event with N number of parameters. This is not an entirely accurate statement but for illustration purposes, in UA you might have one data row per page view or event. In GA4, you have a data row when you first arrive at a page that has five sub-rows, followed by a new row when a session starts which contains five or more sub-rows, followed by a new row when an event occurs like a link click, which contains nine more sub-rows - and so on. The result is that a single visitor can quickly generate hundreds of rows of data.
On one of our accounts, a day with just 1,500 users generated 200,000 rows of data, an average of 133 records per user.
By structuring data collection this way, Google has all but guaranteed that we all need big data - and the only option to store it is in Google BigQuery.
The trouble with this amount of data is obvious when you consider the fact that even Google can no longer process it effectively. Back in the UA days, you could get accurate answers about your analytics data at will. With GA4, Google flat out tells you that they can’t process your real data to give you the answer; instead, they process a small portion of it and run an estimation algorithm to extrapolate the "answer" you see in the GA4 dashboard.
If you want an accurate answer? Your only option is to export the raw data to their BigQuery product and process it from there yourself.
Technical and Financial Ramifications
While they do give some generous free bandwidth, storage, and compute to you to help solve this - you need an extremely technical team to avoid incurring fees. And even then you have to pay somewhere.
Many will decide that it’s simpler to just pay BigQuery and be done with it. With that same modest account mentioned above, which receives an average of 2,500 users per day, the free BigQuery storage will reach its limit after about seven months, at which point it will begin to incur storage costs. You also get 1TB of querying per month for free which is pretty large, however, each query you make has a 10MB per table queried minimum, so it adds up fast if you’re doing data analysis.
With the number of GA properties out there that have been used to free everything with UA, it seems clear that Google is making a play to boost its cloud business by leveraging the popularity of the product.
And it’s working. Google Cloud has just turned a profit for the first time in its existence. This issue is further compounded by the built-in BigQuery connector inside Looker Studio. If you connect your reports directly to the GA4 BigQuery table, it performs an egregious amount of inefficient queries for each component of the report which can add up rapidly. Connecting your report directly to the GA4 data that Google provides via the BigQuery connection is the most obvious and straightforward way to get started - and if you do that, you’ll start to pay the price quickly.
Navigating the Post-UA World
What you’d need to do instead if you wanted to be more efficient is to transform the data into more easily digestible data models, via flattening of transformation. This is far outside of the average customer’s technical capability.
Added to that, you’ll also want a system for pruning historical data out of BigQuery if you want to avoid paying storage fees, but you probably don’t want to lose that data over time. That means you also need a strategy for archiving the data - either in another data warehouse or by transforming it into separate flattened tables that trim back the raw data into only what you need to report on. That is a lot easier said than done.
The orchestration of an ETL (Extract, Transform, Load) system like that is fairly hardcore technical science. Most organizations don’t have that know-how in-house which means they have to pay someone else to do that work as well. The costs can pile up. There are plenty of options of cloud-based tools that can help with this ETL process but they come with substantial time and cost investments.
At Cypress North, we’re working to solve this problem for not only GA4 data but also all of the ad platforms that our clients use along with their CRM and eCommerce platforms to form a unified strategy that can bring back accurate and timely insights for our customers. If you’re interested in discussing a solution, reach out to our team for more information.
Meet the Author
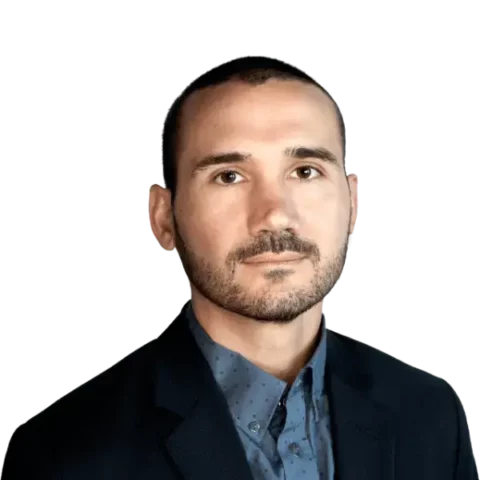
Matthew Mombrea
Matt is our Chief Technology Officer and one of the founders of our agency. He started Cypress North in 2010 with Greg Finn, and now leads our Buffalo office. As the head of our development team, Matt oversees all of our technical strategy and software and systems design efforts.
With more than 19 years of software engineering experience, Matt has the knowledge and expertise to help our clients find solutions that will solve their problems and help them reach their goals. He is dedicated to doing things the right way and finding the right custom solution for each client, all while accounting for long-term maintainability and technical debt.
Matt is a Buffalo native and graduated from St. Bonaventure University, where he studied computer science.
When he’s not at work, Matt enjoys spending time with his kids and his dog. He also likes to golf, snowboard, and roast coffee.